
The questions of whether adjusting for baseline covariates is justified or even desirable is indeed a recurrent one with a long history in the context of randomised controlled trials. The underlying rationale is similar to that for the standard practice of adjusting for baseline covariates in models of the outcome in randomised controlled trials, where the prognostic factors Z and the (randomised) treatment X can be seen as forming a v-structure with the outcome Y as the collider. Intuitively we would expect that the extent by which estimates differ will depend on the strength of the relationship between Y and Z. The estimator using raw conditionals is therefore the same whether the edge from Z → Y is present, while the approach using marginalisation would give different estimates for the two cases. Since Y would then be independent of Z, the marginalisation would reduce to the raw conditionals. It is instructive to also consider the DAG with the edge from Z → Y deleted. To answer the question we compute the variance, for finite sample sizes, of the two different estimators corresponding to the implicit and explicit marginalisation as outlined earlier.

Since we see that adjustment by Z is valid, but not necessary, it is natural to ask whether the two estimators differ in terms of precision.
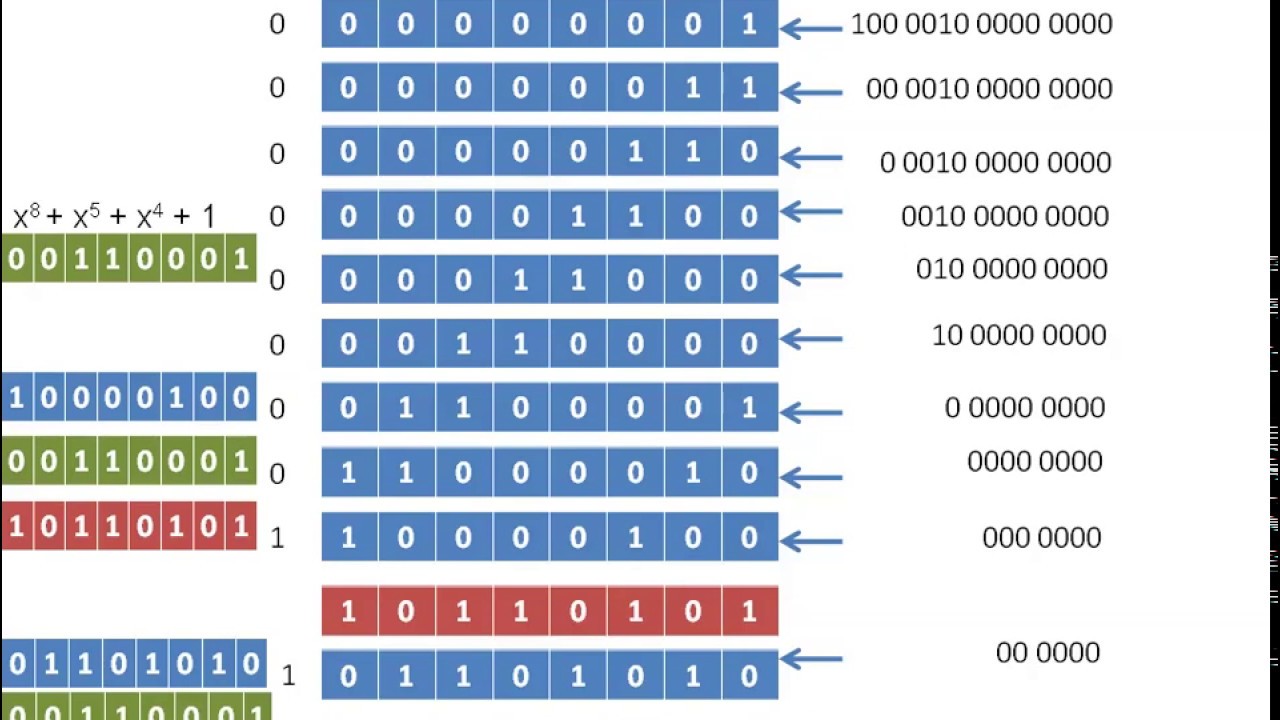
With the latter equality justified by structural and invariance properties and also in agreement with the standardisation formula in equation ( 1). (7) p ( Y ∣ do ( X ) ) = ∑ Z p ( Y ∣ do ( X ), Z ) p ( Z ∣ do ( X ) ) = ∑ Z p ( Y ∣ X, Z ) p ( Z ) , For binary data and relatively small networks, one can explicitly marginalise over the remaining nodes in the DAG and its parameters to derive interventional distributions as follows: To explore the precision of causal estimators for non-linear models, we consider the simplest such case: a DAG with three nodes of binary variables organised in a v-structure with the outcome Y of interest as a collider with parents Z and X ( Figure 1) and with the latter being the exposure whose effect we wish to estimate. Even more recently, this has been extended to non-parametric estimators, and the asymptotically optimal set has been further characterised. In evaluating the variance of different estimators, the remarkable result that the asymptotically optimal adjustment set can be determined solely based on graphical criteria regardless of the edge coefficients has recently been obtained. Although all valid adjustment sets provide consistent estimators of the causal effects, for finite-sized data, different adjustment sets can lead to different numerical estimates, and with different precisions. The set of parents of X always satisfies the back-door criterion and is therefore a valid adjustment set, but there may be many more depending on the graphical structure of the DAG. This also holds for linear non-Gaussian causal models. įor linear Gaussian models, the marginalisation can be simply estimated by regressing Y on X and Z and extracting the coefficient of X, hence the naming of “adjustment” sets. (1) p ( Y ∣ do X ) = p ( Y ∣ X ) if Z = ∅ ∫ z p ( Y ∣ X, z ) p ( z ) d z otherwise. As a practical consequence, the adjustment set selection needs to account for the relative magnitude of the relationships between variables with respect to the sample size and cannot rely on purely graphical criteria. Further, by going beyond leading-order asymptotics, we show that there are parameter regimes where the set with the asymptotically optimal variance does depend on the edge coefficients, a result that is not captured by the recent leading-order developments for general causal models. We explicitly compute and compare the variance of the two possible different causal estimators. To investigate the extent of this variability, we consider the simplest non-trivial non-linear model of a v-structure on three nodes for binary data.
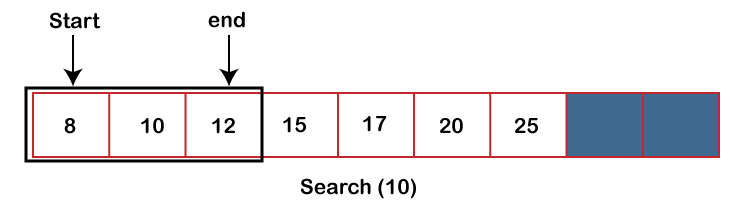
However, in practice, with finite data, estimators built on different sets may display different precisions. Depending on the causal structure of the mechanism under study, there may be different adjustment sets, equally valid from a theoretical perspective, leading to identical causal effects. Adjusting for covariates is a well-established method to estimate the total causal effect of an exposure variable on an outcome of interest.
